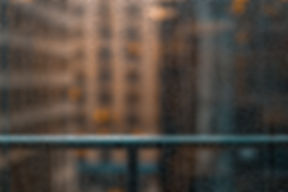
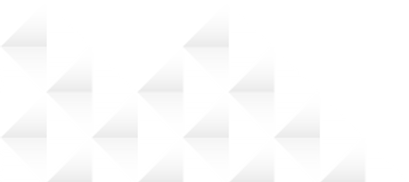
GRACE Soil Moisture Retrievals
This dataset includes monthly volumetric surface (0-5 cm) soil moisture data from May 2002 to May 2017, which were retrieved from the NASA Gravity Recovery and Climate Experiment (GRACE) satellite observations. The GRACE terrestrial water storage (TWS) data were fed into an analytical solution to the linearzied Richards' equation to yield these soil moisture values. The data provide new long-term soil moisture information beyond what can be retrieved from the microwave satellites such as the NASA Soil Moisture Active Passive (SMAP), especially in densely vegetated areas where the microwave signal is decoupled from soil moisture. For more details about the methodology and dataset, please see: Sadeghi, M., Gao, L., Ebtehaj, A., Wigneron, J. P., Crow, W. T., Reager, J. T., & Warrick, A. W. (2020). Retrieving global surface soil moisture from GRACE satellite gravity data. Journal of Hydrology, 584, 124717. https://doi.org/10.1016/j.jhydrol.2020.124717.
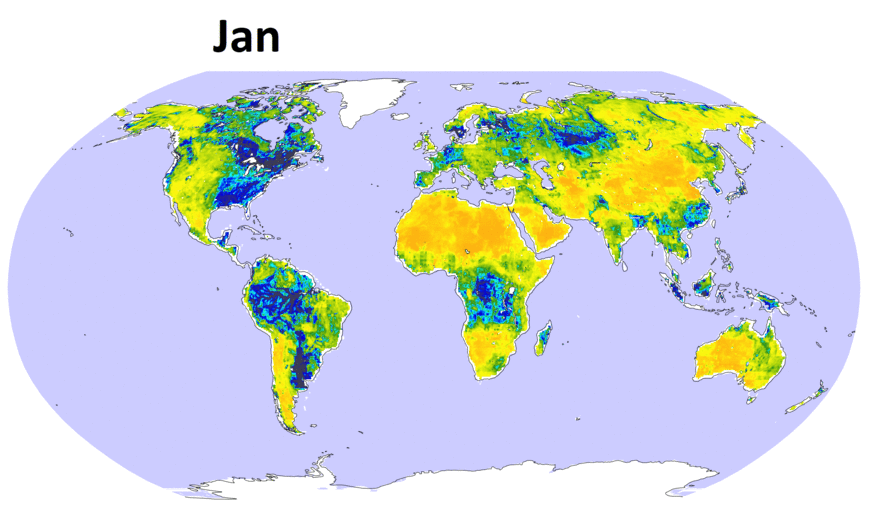
High-resolution Passive Microwave Soil Moisture Retrievals
Global monitoring of soil moisture (SM) and vegetation biomass from space is important for improved land and weather forecasts understanding of water, energy , and carbon cycles as well as improved management of water and food resources. Today spaceborne platforms, especially the ESA's Soil Moisture and Ocean Salinity (SMOS, 2009-present) and NASA's Soil Moisture Active Passive (SMAP, 2015-present) satellites provide an unprecedented opportunity to estimate SM of the top 5-cm soil layer and vegetation optical depth (VOD) every 2 to 3 days globally. These satellite missions rely on L-band (1.4 GHz) radiometry and enable all-weather SM sensing under canopies with vegetation water content of less than 5 kilogram per square meter.
A series of new algorithms called combined constrained multi-channel algorithm (C-CMCA) is developed for simultaneous retrieval of soil moisture (SM) and vegetation optical depth (VOD) in L-band with improved resolution. Unlike widely used algorithms, the new approach optimally fuses multiple sources of surface temperatures into the inversion process and confines the retrievals to their physical and climatological range of the retrievals and paves the way to account for the slow changes of VOD through a smoothing-norm regularization. Through controlled numerical experiments and continental implementation using SMAP observations we consistently show that C-CMCA can can increase the resolution of SM and VOD retrievals. Analysis of the results demonstrates that, without increasing the native resolution of radiometric observations, the information content of the a priori constraints cannot only improve resolutions of the retrievals but also make them robust to the background water contamination in the vicinity of coastal zones and over lowland floodplains. For example, over Florida peninsula, the annual SM root mean squares error and bias are reduced by more than 65%.
A zoom in of the SM retrievals over Florida on 02/15/2016 from SMAP, C-CMCA as well as the soil texture, MODIS derived VOD, and daily time series of spatial mean values of SM retrievals in 2016. The comparisons are provided over days when at least 10 reference ISMN SM values are available. The soil texture labels denote sand (1), loamy sand (2), sandy loam (3), silt loam (4), silt (5), loam (6), sandy clay loam (7), silty clay loam (8), clay loam (9), sandy loam (10), silty clay (11), clay (13), and others (13).
A zoom in of the monthly averaged SM retrievals in February 2016, soil texture, MODIS VOD, and one year time series of spatially-averaged SM in 2016 over the alluvial plain of the lower Mississippi basin. The spatially-averaged SMAP and C-CMCA daily SM values with VOD less than 0.5 [-] are compared with reference gauges for days when at least ten gauges are providing measurements. Please move the slider to the left to see the results over the lower Mississippi basin.
​
Please see Ebtehaj and Bras (2019) and Gao et al., (2020).
ftp://ebtehaj.safl.umn.edu/Codes/CMCA/ https://ebtehaj.safl.umn.edu/Codes/CMCA/
ftp://ebtehaj.safl.umn.edu/CodeS/C_CMCA/ https://ebtehaj.safl.umn.edu/CodeS/C_CMCA/
Precipitation Passive Microwave Retrieval
The upwelling earth radiation in microwave bands contains spectral signatures that allow us to measure global precipitation from space. In the past few years, our team has developed a new passive retrieval algorithm to obtain improved estimates of precipitation from space using passive radiometric measurements provided by the Tropical Rainfall Measuring Mission (TRMM) and Global Precipitation Measurement (GPM) satellites.
Our retrieval algorithm, called ShARP (Shrunken Locally Linear Embedding for Passive Microwave Retrieval of Precipitation), relies on manifold learning via Bayesian sparse approximation and promises improved passive retrieval of precipitation, especially over land and at the vicinity of coastlines. Some key outcomes of the ShARP retrievals are shown in the figures below in comparison with the standard TRMM-2A12 passive retrieval products during the entire calendar year 2013. It can be seen that ShARP promises improved retrievals over arid and semi-arid (e.g., Sahara Desert) regions and mitigates the commonly observed over-estimation of rainfall over snow-covered land surface (e.g., Tibetan highlands).
A software package, using MATLAB computing language, has been developed. The package can be freely downloaded and used for research purposes.The codes are primarily tested using MATLAB (R), 2014-15. The package requires the "signal processing" and "optimization" toolboxes (see, the readme file). The current version of the algorithm is memory intensive as it uses spectral and rainfall dictionaries containing a large number of brightness temperatures and their coincident rainfall profiles. A random access memory of at least 16 GB is recommended depending on the size of the employed dictionaries for the current version. An ongoing effort is underway to produce a parallel version of the algorithm to make more efficient. Please read more ...
Cyclone Sidr, 2007
Hurricane danielle, 2010
A squall line, GA, 2013
Top panel shows all active retrievals of the TRMM overpasses in 2013. The image shows mean values of more than 5200 of the TRMM overpasses. Active PR-retrievals are considered as a relative reference in our comparison.
Bottom panel compares the standard 2A12 passive retrievals with the results by the ShARP algorithm. It is shown that how the ShARP algorithm can reproduce the radar active products from passive observations of multi-spectral brightness temperature values. As is clear, ShARP promises improved retrievals over arid and semi-arid climate (e.g., Sahara Dessert) and reduced retrieval biases over cold mountainous regimes covered with snow (e.g., Himalayas and Tibetan Highlands).
Compressive Earth Observatory
The Compressive Earth Observatory (CEO) is a new conceptual framework that uses Compressive Sensing (CS) theory for the efficient estimation and sampling of land atmosphere state variables and fluxes from space. In a paper, published in Geophysical Research Letters, (Ebtehaj et al., 2015) using the retrievals of Atmospheric Infrared Sounder (AIRS) on board of NASA’s Aqua satellite, we demonstrated that: 1) the geophysical fields such as temperature and moisture fields are sparse in the wavelet domain, throughout the depth of atmosphere and 2) using a small set (30%) of random samples of temperature and moisture fields, the CS theory enables us to recover the entire field with high degree of accuracy. The main messages are: a) we may be able to design a next generation of sensors that allow to collect a smaller number of samples without compromising the accuracy of the earth observatory systems. b) With current sensing protocols, we may be able to design compatible and operationally viable random sampling schemes that enable significant reduction of the sampling density from space, leading to increased life span of the spacecraft, reduction in latency time of data transfer, and speedy retrievals for early warning systems.
Iterative reconstruction of an eight-day (2/10-10/01/2002) average surface skin temperature [Kelvin] using CS theory and data provided by the NASA's AIRS instrument. The down-sampled field only contains 35% of randomly chosen pixels. The energy of the reconstruction error is less than 0.01% of the total energy of the original temperature field. This reconstruction uses a stationary wavelet transform as the sparsifying operator and relies on the Fast Iterative Shrinkage Thresholding (FISTA) for solving the CS problem. Please read more...